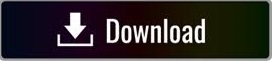
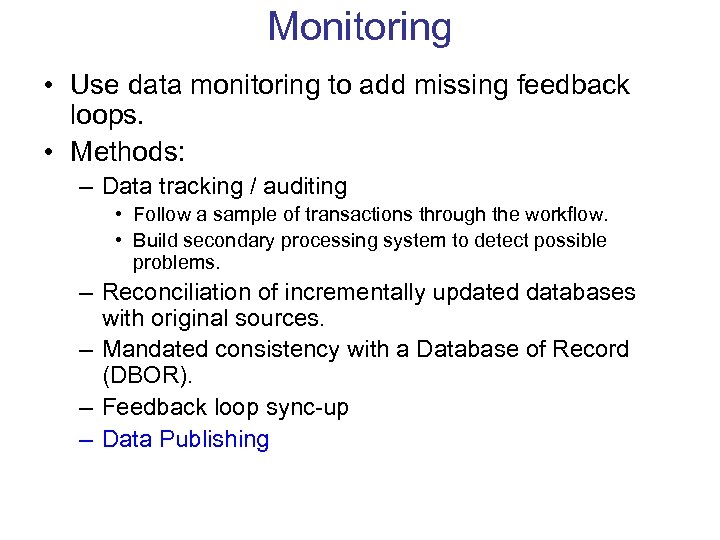
In addition, we can simultaneously combine information from multiple data sources by expressing multiple datasets in terms of the same low dimensional space. By reducing the covariate dimension we aim to diminish the risk of overfitting and increase the robustness and accuracy with which we infer relationships between covariates and survival outcomes. The com- bined model offers a flexible non-linear probabilistic method of detecting and extracting any intrinsic low dimensional structure from high dimensional data. Here we propose a novel method of extracting a low dimensional rep- resentation of covariates in survival data by combining the popular Gaussian Process Latent Variable Model (GPLVM) with a Weibull Proportional Hazards Model (WPHM). The analysis of high dimensional survival data is challenging, primarily due to the problem of overfitting which occurs when spurious relationships are inferred from data that subsequently fail to exist in test data.
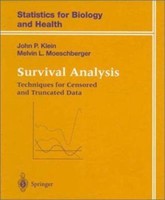
Simulation studies suggest that in some cases assuming dependence can lead to more accurate predictions. By tuning one of the model parameters we can control the extent to which the multiple outputs (the time-to-event for each risk) are dependent thus allowing the specification of correlated risks.

We then apply multiple output GP regression, which can handle multiple potentially correlated outputs for each input, to competing risks survival data where multiple event types can occur. We apply our approach to both simulated and experimental data. It is straightforward to include various types and combinations of censored and truncated observations. Our proposed model belongs to the class of accelerated failure time models where we focus on directly characterising the relationship between covariates and event times without any explicit assumptions on what form the hazard rates take. Many existing methods, such as the ubiquitous Cox proportional hazards model, focus primarily on the hazard rate which is typically assumed to take some parametric or semi-parametric form. This allows for highly flexible inference of non-linear relationships between covariates and event times. In this context, the covariates are regarded as the `inputs' and the event times are the `outputs'. We apply Gaussian process (GP) regression, which provides a powerful non-parametric probabilistic method of relating inputs to outputs, to survival data consisting of time-to-event and covariate measurements.
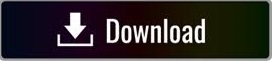